التنبؤ بمخاطرة أسعار الصرف باستخدام الشبكة العصبونية-دراسة تطبيقية
DOI:
https://doi.org/10.31272/jae.i129.43الكلمات المفتاحية:
الشبكة العصبونية، مخاطرة أسعار الصرف ، التنبؤالملخص
تتميز تقنية الشبكات العصبونية (Neural Networks) بقدرتها على معالجة المدخلات ومعطيات الواقع وتجاوز التقنيات التقليدية في تفسير سلوك الظواهر، مما جعلها مثار اهتمام العديد من الباحثين في المجالات المختلفة. يهدف البحث الى الإفادة من القدرات المتميزة للشبكة العصبونية عند استخدامها في اتخاذ قرارات الإستثمار لاسيما في مجال العملات كونه أحد مجالات الإستثمار المهمة سواء على مستوى الأفراد أم المؤسسات المالية بهدف تحقيق الأرباح، والتي تعتمد أساساً على التنبؤ بتحرك أسعار العملات صعوداً أو نزولاً. تم استخدام ثلاث أنواع من تقنيات الشبكات العصبونية وهي: تقنية الشبكة العصبية الشعاعية المحدثة (Modified Radial Basis Function(MRBF)) وتقنية الشبكة العصبية متعددة الطبقات (multi-layer perception (MLP)) وأخيراً تقنية الشبكة العصبية الاصطناعية(artificial neural network (ANN)) لتحقيق أهداف البحث. تم إجراء التحليل باستخدام معلومات شهرية عن سعر صرف اليورو مقابل الدولار الأمريكي للمدة من 1/1/2015 لغاية 31/12/2020 ، و تم استخدام برنامج (MATLAB, V.5.2.0) لمعالجة بيانات الإدخال وبالتالي الحصول على نتائج البحث. توصل البحث الى ان استخدام الشبكة العصبونية (ANN) يعطي دقة كبيرة في التنبؤ بالتحركات المستقبلية للعملة عينة البحث قياساً بالتقنيات الأخرى المستخدمة حسب معيار المقارنة RMSE، الأمر الذي يقلل من مخاطرة الإستثمار في العملات وبالتالي زيادة العوائد.
المراجع
1- الجراح ، نوال و الحكاك، ندى (2013)، "إستخدام الطرق الهجينة في التنبؤ لسعر الصرف للدولار الأمريكي مقابل الدينار العراقي"، مجلة كلية بغداد للعلوم الاقتصادية الجامعة ، العدد 34، 359-380.
2- سلمان، ماهر محسن، "التنبؤ بإحتمالات تغير سعر صرف الدينار العراقي مقابل الدولار الأمريكي بإستعمال سلاسل مارآوف للفترة (2008-2014)" ، البنك المركزي العراقي / المديرية العامة للإحصاء والأبحاث / قسم بحوث السوق المالية .
3- Al-Momani, Riad & R. Gharaibeh, Mohammad. (2008). Foreign exchange risk management practices by Jordanian nonfinancial firms. Journal of Derivatives & Hedge Funds. Palgrave Macmillan .Vol. 14 , Nos. 3/4, pp. 198–221. DOI: https://doi.org/10.1057/jdhf.2008.16
4- Bodie, Z. Kane, A. and Marcus, A. (2011). Investment and Portfolio Management. 9E McGraw-Hill. USA.
5- Chandrasekara, N. V & Tilakaratne, C. D. (2009). Forecasting exchange rates using Artificial Neural Networks, Sri Lankan Journal of Applied Statistics, 10 (2009): 187 – 201.
6- Chandrasekara, N.V. & Tilakaratne , C.D. (2014), “ Forecasting Exchange Rates using Artificial Neural Networks”, Sri Lankan Journal of Applied Statistics, No.10, pp. 187-201.
7- Cochetti, S. Schoenholtz, K.and Fackler, James. (2011). Money, Banking and financial market. 3E McGraw-Hill. USA.
8- Cohen. B. 2009. Future of the reserve currency. Finance and Development. September(2009).
9- Hallgren ,Anna, Ljung, Nils-Petter & Temler Linda . (2006) .Swedbank, Bachelor Dissertation, Department of Business Studies. pp. 1-85.
10- https://sa.investing.com/currencies/eur-usd-historical-data
11- Jacque, Laurent L. (1996). Management and Control of Foreign Exchange Risk. Kluwer Academic Publishers. Currency Risk Management-A case study of two Swedish mid-corps DOI: https://doi.org/10.1007/978-94-009-1806-1
12- James, R. (2012). Currency Wars: The Making of the Next Global Crisis. Penguing Group. USA.
13- Jordan, B. Westerfield, R. and Ross, S. (2011). Corporate Finance essential. 7E. McGraw-Hill. U.S.A.
14- Kumar, P. and Walia, E. (2006). Cash Forecasting:An Application of Artificial neural networks in finance. International Journal of Computer Science & Application 111 (1).
15- Lean Yu , Shouyang Wang & Kin Keung Lai, (2007). "Foreign-Exchange-Rate Forecasting With Artificial Neural Networks," International Series in Operations Research and Management Science, Springer, number 978-0-387-71720-3.
16- Madhumathi, R. and Ranganatham. M. (2012). Derivatives and Risk management. Pearson. Delhi.
17- Maditinos, Dimitrios, Chatzoglou and Prodromos. (2002). The Use of neural networks in forecasting. Review of Economic Science 6 .
18- Madura, J. and .Fox, R. (2007). International financial management. Thomson Learning. UK.
19- Mark, B. (2008). The Social Atom. Al.dar Masriah. Al-lubnaniah. Egypt.
20- Michael G. Papaioannou. (2006) . Exchange Rate Risk Measurement and Management: Issues and Approaches for Firms. International Monetary Fund (IMF) . No. 06/255. pp. 1-20. DOI: https://doi.org/10.5089/9781451865158.001
21- Moghaddam, A. Moghaddam. M.and Esfandyari, M. (2016). Stock market index prediction using artificial neural network. Journal of Economic. Finance. Administrative Science. Wo.21. DOI: https://doi.org/10.1016/j.jefas.2016.07.002
22- Nasution, Bona Patria & Agah, Arvin ,(2000), “Currency Exchange Rate Forecasting with Neural Networks”, Journal of Intelligent Systems Vol. 10, No. 3,pp. 219-253. DOI: https://doi.org/10.1515/JISYS.2000.10.3.219
23- Nguyen, Joseph,(2019), “4 Ways to Forecast Currency Exchange Rates, Investopedia, pp. 6-10, Link : https://www.investopedia.com/articles/forex/11/4-ways-to-forecast-exchange-rates.asp.
24- Philip, Adewole Adetunji, Taofiki, Akinwale Adio & Bidemi, Akintomide Ayo, (2011) “Artificial Neural Network Model for Forecasting Foreign Exchange Rate”, World of Computer Science and Information Technology Journal (WCSIT) ISSN: 2221-0741 Vol. 1, No. 3,pp.110-118.
25- Queen, Abdulkadhim M., Alborgeef, Kafaa Ali, & Jebur, Tuka Kareem, (2020), “Predicting exchange rate future movements by Applying Modified Genetic Radial Basis Function Neural Network, Journal of Xi'an University of Architecture & Technology, Volume XII, Issue IV, pp.6121-6138.
26- Ross, S. Westerfield, R. Jordan, B. and Biey, J. (2013). Corporate Finance. McGraw – Hill. U.S.A.
27- Rowland, Zuzana, Lazaroiu, George & Podhorská, Ivana,(2020) ,“Use of Neural Networks to Accommodate Seasonal Fluctuations When Equalizing Time Series for the CZK/RMB Exchange Rate”, MDPI stays neutral with regard to jurisdictional claims in published maps and institutional affiliations. DOI: https://doi.org/10.3390/risks9010001
28- Sollis, R. (2012). Empirical finance for finance and Banking. John Wiley Sons Ltd. U.K.
29- SUN, Yawen (2005), “Exchange Rate Forecasting with An Artificial Neural Network Model: Can We Beat a Random Walk Model?’, A thesis submitted in partial fulfilment of the requirements for the degree of Master of Commerce and Management (M.C.M.), Lincoln University .
30- Tan, C. (2014). An Artificial neural network primer with financial application examples in financial distress predictions and foreign exchange hybrid trading system. Data Science Association. Paper Academic.
31- Tavish ,Srivastava,(2014), “How does Artificial Neural Network (ANN)algorithm work? Simplified, Link: https://www.analyticsvidhya.com/blog/2014/10/ann-work-simplified.
32- Thawornwong, S.and Enke, D. (2004). The adaptive selection of financial and economic variables for use with artificial neural network. Neuro Computing Wo:56. DOI: https://doi.org/10.1016/j.neucom.2003.05.001
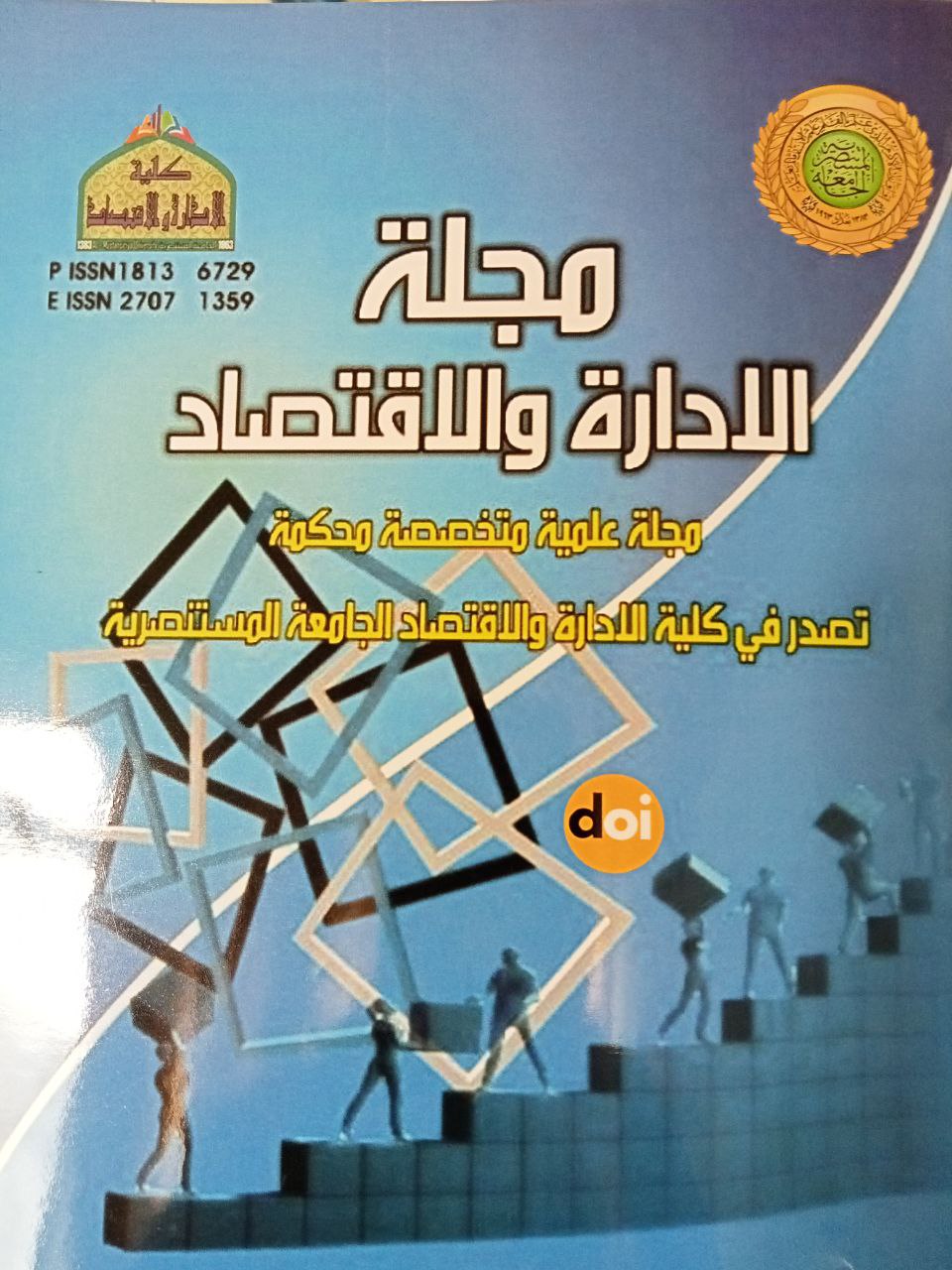
التنزيلات
منشور
إصدار
القسم
الرخصة
الحقوق الفكرية (c) 2022 مجلة الإدارة والاقتصاد

هذا العمل مرخص بموجب Creative Commons Attribution 4.0 International License.
مجلة الإدارة والاقتصاد هي مجلة مفتوحة المصدر حيث تكون جميع محتوياتها مجانية. تخضع مقالات هذه المجلة لشروط ترخيص المشاع الإبداعي المنسوب إلى المؤلف (CC-BY 4.0) (https://creativecommons.org/licenses/by/4.0/legalcode) الذي يسمح للمرخص لهم دون قيود بالبحث عن النص الكامل للمقالات أو تنزيله أو مشاركته أو توزيعه أو طباعته أو ربطه به، وفحصه للفهرسة وإعادة إنتاج أي وسيلة للمقالات بشرط أن ينسبوا إلى المؤلفين الفضل في ذلك (الاستشهاد). تسمح المجلة للمؤلفين بالاحتفاظ بحقوق الطبع والنشر لمقالهم المنشور.
. Creative Commons-Attribution (BY)