Estimating the parameters of the GARCH model for the Polya distribution with a practical application
DOI:
https://doi.org/10.31272/jae.i142.1035Keywords:
autoregression conditional on heterogeneity of variance, maximum likelihood method (MLE), Polya distributionAbstract
The research aims to study time series with high volatility (volatility) , in which the problem of autoregressive conditional on the heterogeneity of variance in the case of intermittent distributions , that is when its observations have integer values . The INGARCH model was studied when the series follows the Polya distribution , which was Studying the model theoretically and practically , and then estimating the model parameters using the maximum likelihood method (MLE) , and in the applied aspect , data on the number of transactions of the Sumer Commercial Bank in the Iraqi Stock Exchange was used , where the presence of the ARCH effect was tested using the Ljung - Box Test and (ARCH Test) It became clear from the two tests that the data suffers from the problem of heterogeneity of variance (ARCH) , and finally it was concluded that the model that explains the fluctuations in the number of transactions of Sumer Commercial Bank is the INGARCH (2.0) model , based on the results of the two standards AIC and BIC .
References
- علي ياسين غني البدراوي (2015) ، " اثر التوزيع غير الطبيعي لحدود الخطأ العشوائي في تقدير معلمات بعض نماذج ARMA-GARCH مع تطبيق" اطروحة دكتوراه , الجامعة المستنصرية .
- Bollerslev T. (1987), “A Conditionally Heteroskedastic Time Series Model for Speculative Prices and Rates of Return” Review of Economics and Statistics, Vol. 69, pp 542-547.
- Engle R.F. (1982), "Autoregressive Conditional Heteroscedasticity with Estimates of the Variance of United Kingdom Inflation" Econometrica, Vol. 50, No.4, pp 987-1007.
- Ferland, R., Latour A., & Oraichi D. (2006), "Integer–Valued GARCH Processes" Journal of Time Series Analysis, Vol. 27, pp 923–942.
- Francq, C. and J.-M. Zakoian, GARCH models: structure, statistical inference and financial applications. 2011: John Wiley & Sons .
- Tripathy, N. and A. Garg, Forecasting stock market volatility: Evidence from six emerging markets. Journal of International Business and Economy, 2013. 14(2): p. 69-93.
- Tsay R.S. (2005), Analysis of Financial Time Series, Second Edition, John Wiley & Sons, Inc., Hoboken, New Jersey .
- Walck C., & Fysikum (2007), "Hand-book on Statistical Distributions for Experimentalists" University of Stockholm.
- Weiß C.H. (2010), "INARCH(1) Processes: Higher-Order Moments and Jumps" Statistics and Probability Letters, Vol. 80, pp 1771-1780.
- Zhu F. (2011), " A Negative Binomial Integer-Valued GARCH model" Journal of Time Series Analysis, Vol. 32, pp 54–67.
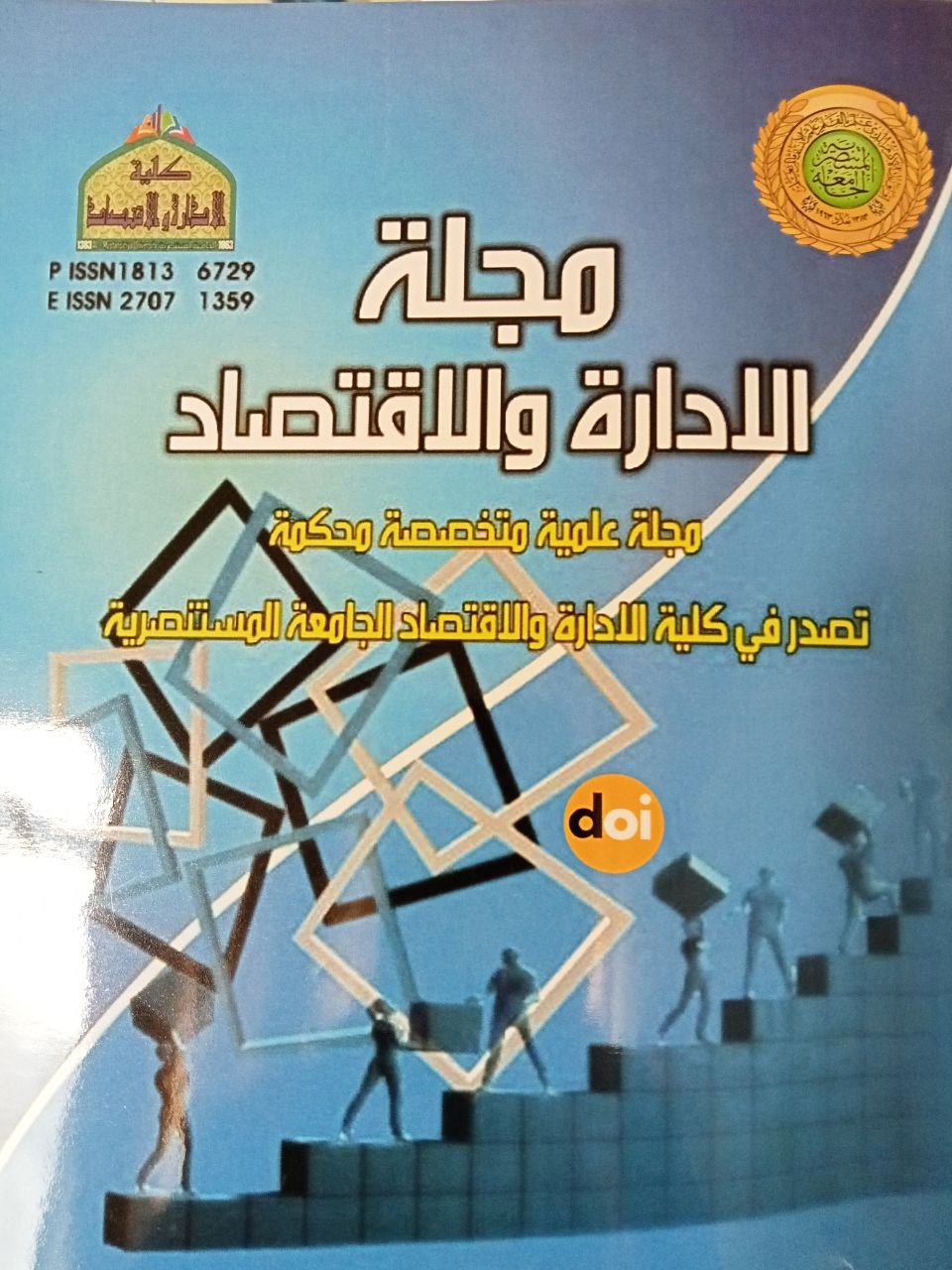