High-Dimensional variance matrix estimation using the OGK genetic algorithm
DOI:
https://doi.org/10.31272/jae.i147.1309Keywords:
معلمة التنظيم , الحد الادنى لمحدد التباين المنتظم , مصفوفة الهدف , مهلنوبسAbstract
This research estimated a high-dimensional variance matrix when the number of variables was more significant than the number of observations. The OGK genetic algorithm was applied to find the variance matrix. A modification for the genetic algorithm OGK was proposed depending on the regular parameter ρ and the target matrix T, and It was called the (Orthogonalized Regularized Gnanadesikan – Kettenring ) it can be written briefly ( ORGK ), The data was taken from four stations representing the monthly rates for a group of polluted for air the gases for one year. The monthly rates were measured for four types of gases (Methane gas , Carbon Monoxide gas CO , Nitrous gas , Sulfur Dioxide gas ) . In this study, a comparison was made between the genetic algorithm OGK, ORGK and MRCD by finding the determinant of the covariance matrix and identifying the most polluting gasses. Carbon Monoxide CO was the main cause of pollution. And the ORGK algorithm dependent of the regular parameter and target matrix, it has a clear effect in obtaining the lowest determinant of the covariance matrix, which is called (Orthogonalized Regularized Gnanadesikan – Kettenring ) ORGK
References
[1] Clifferd lam .High Dimensional Covariance Matrix Estimation.Department of statistics.London School. http://stats.lse.ac.uk.
[2] Fatimah Abdul – Hammeed and Mohammad Huseen Abdul – Hammeed,(2011),” Estimated between the two-stage summation shrinkage for the variance of a normal distribution and for equal sizes of the two samples”,Baghdad science journal,(Jun.2011),No1009.
[3] Fatimah Abdul – Hammeed and Sabah Manfi ,(2016),” Compared with genetic algorithm Fast-MCD-Nested Extension and neural network multilayer Back propagation”, JOURNAL OF ECONOMIC&ADMINISTRATIVE SCIENCE ,(Jun.2016),No 22(89),pp.381-395.
[4] Gnanadesikan,R.and Kettection,j,( 1972),”Roubust estimates,residuals,and outlier detection with multiresponse data.Biometrics”, 28,pp. 81-124 .
[5] Hubert,M.and Debruyne,M,( 2010),”Minimum Covariance Determinant.Wiley Interdisciplinary Reviews:Computional Statistics” ,2, 36-34.
[6] Jan Kalina,jurjen Duintjer Tebbens,and Anna Sehlenker .Robustness of High Dimensional Data Mining.https://www.sementisy scholar.org.
[7] Ledoit , O. and Wolf ,M,( 2019),”Quadratic Shrinkage for Large Covariance Matrices “,University of Zurich .(November.2019).
[8] Ledoit , O. and Wolf ,M,(2004),”A well-conditioned estimator for large-dimensional covariance matrices”, Journal of Multivariate Analysis,88(2),pp.365-411. DOI: https://doi.org/10.1016/S0047-259X(03)00096-4
[9] Mia.Hert.and Paterj.Rousseeuw.and Tim Verdonck,(2011),”Adeterministic algorithm for robust Location and scatter”,pp.journal of computational(2012).
[10] Peter j.Rousseeuw,Steven Vandumffel,Tim Verdonck,( 2018),”The Minimum Regularized Covariance Determinant Estimator”,ar Xiv:1701.07086v3(November .2018) ,29.
[11] Rousseeuw,P.and Van Driessen,K,( 1999),”Afast algorithm for the Minimum Covariance Determinant estimator”, Technometrics 41,pp. 212-223.
[12] Virgile Fritsch,Gael varoquaux,Thyreau Benjamin,Jean-Baptiste poline,Bertrand Thirion,(2011),”Detecting outlying subjects in High-Dimensional Neuroimaging Data sets with Regularized Minimum Covariance Determinant”,HAL .(sep. 2011),27. DOI: https://doi.org/10.1007/978-3-642-23626-6_33
[13] Yilun,Ami wiesel,AlfredO,( 2010),”HeroIII.Robust Shrinkage Estimtion of high Dimensional Covariance Matrices”,arXiv:1009.5331v1[stat.ME].27(sep.2010).
[14] Zongliang Hu ,Kai Dong , Wenlin Dai and Tiejan Tong,( 2017),”Acomparison of methods for Estimating the Determinent of High-Dimensional Covariance Matrix”.(August.2017),16.
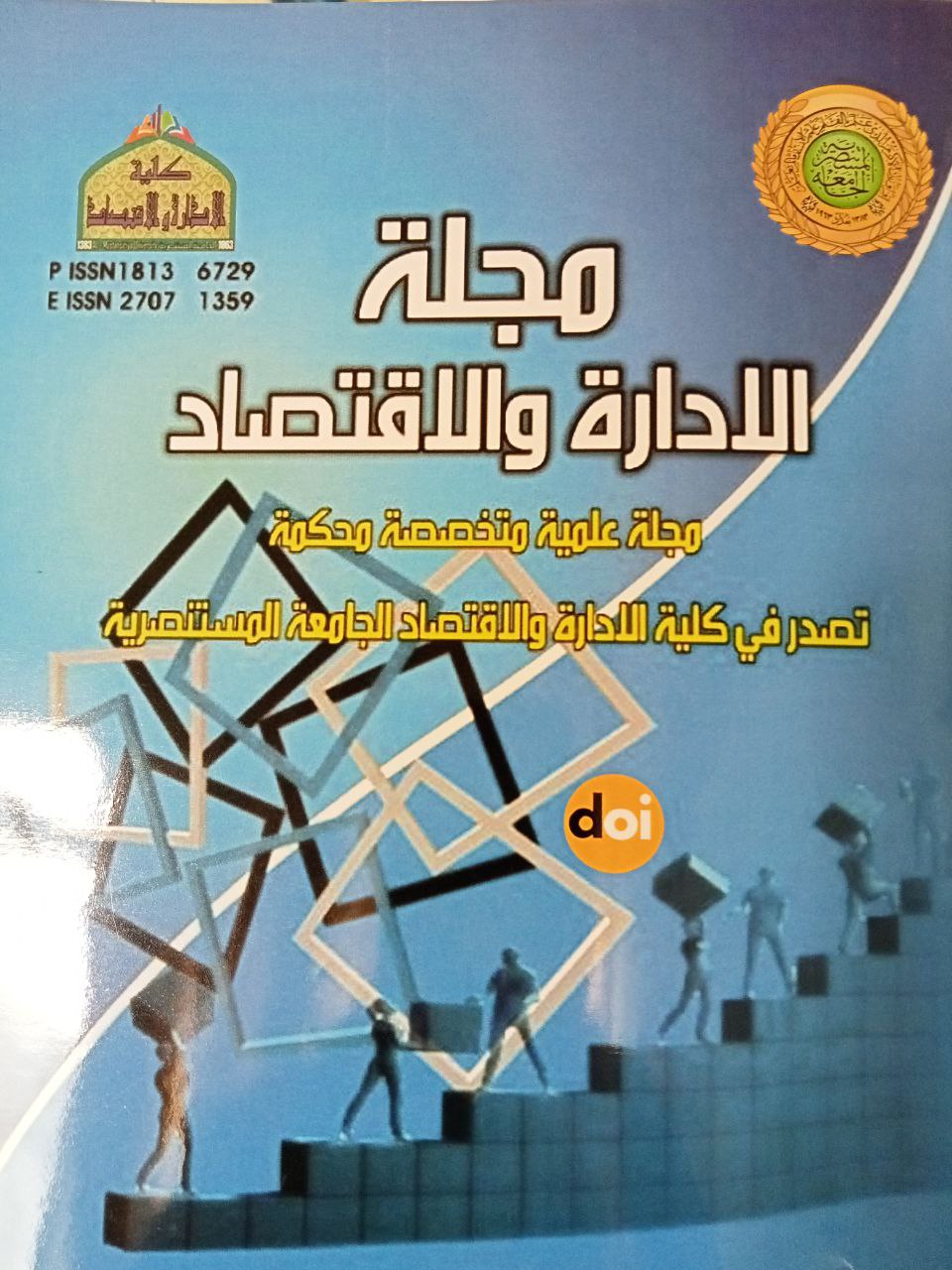
Downloads
Published
Issue
Section
Categories
License
Copyright (c) 2025 أ.د.فاطمة عبد الحميد جواد البيرماني

This work is licensed under a Creative Commons Attribution 4.0 International License.
The journal of Administration & Economics is an open- access journal that all contents are free of charge. Articles of this journal are licensed under the terms of the Creative Commons Attribution International Public License CC-BY 4.0 (https://creativecommons.org/licenses/by/4.0/legalcode) that licensees are unrestrictly allowedto search, download, share, distribute, print, or link to the full text of the articles, crawl them for indexing and reproduce any medium of the articles provided that they give the author(s) proper credits (citation). The journal allows the author(s) to retain the copyright of their published article.
Creative Commons-Attribution (BY)