Prediction using RCPAR cyclic autoregressive model
DOI:
https://doi.org/10.31272/jae.i138.1118Keywords:
RCPAR (1) model, PAR (1) model, FGNLS estimatorAbstract
The periodic autoregressive model with first-order random coefficients RCPAR (1) is one of the models that is based on imposing the best description of seasonal variation and variances by allowing the parameters in the autoregressive to influence the seasons. The researchers (Franses and Papp, 2011) are the first to present this model. It can be easily used for high-frequency seasonal data that repeats in different patterns and works to reduce the number of periodic parameters to obtain sufficient degrees of freedom, and then to reach efficient estimates and accurate predictions. The FGNLS method was applied in estimating the model parameters, as well as comparing the estimation results with the unconstrained PAR (1) cyclic model and then using the estimated model to predict the quantities of liquid gas consumed globally measured (in millions of cubic meters). It was found that the parameters estimated by the (FGNLS) method of the RCPAR (1) model were close to the estimates of the unrestricted PAR (1) model, with the studied model keeping the least number of parameters.
References
Abdelhakim Aknouche,A and Guerbyenne,H.(2009).” Periodic stationarity of random coefficient periodic autoregressions.”, Statistics and Probability Letters 79, pp. 990-996.
Abdullah, N.A., Mohamed ,I. , Peiris, S. & Azizan ,A.A.(2011). ” A New Iterative Procedure for Estimation of RCA Parameters Based on Estimating Functions.”, Applied Mathematical Sciences, Vol. 5, No. 4, pp. 193 - 202
Araveeporn,A.(2020).” Comparing Parameter Estimation of Random Coefficient Autoregressive Model by Frequentist, Method.”, Mathematics 8(1),62.
Basawa ,I.R, Lund ,R. and Shao, Q.(2004).” First - order Seasonal Autoregressive Processes with Periodically Varying Parameters.”, Statistics and Probability Letters, 67, pp. 299-306.
Bloomfield, P., H. L. Hurd and R. B. Lund (1994), Periodic correlation in stratospheric ozone data, Journal of Time Series Analysis 15, 127–150.
Franses,P.H. and Paap,R.(2011).” Random-coefficient periodic autoregressions.”, Statistica Neerlandica , Vol. 65, No.1, pp. 101–115.
Herwartz, H. (1999), Performance of periodic time series models in forecasting, Empirical Economics 24, 471–301.
Jones, R. H. and W. M. Brelsford (1967), Time series with periodic structure, Biometrika 54, 403–407.
Osborn, D. R. and J. P. Smith (1989), The performance of periodic autoregressive models in forecasting seasonal U.K. consumption, Journal of Business and Economic Statistics 7, 117–127.
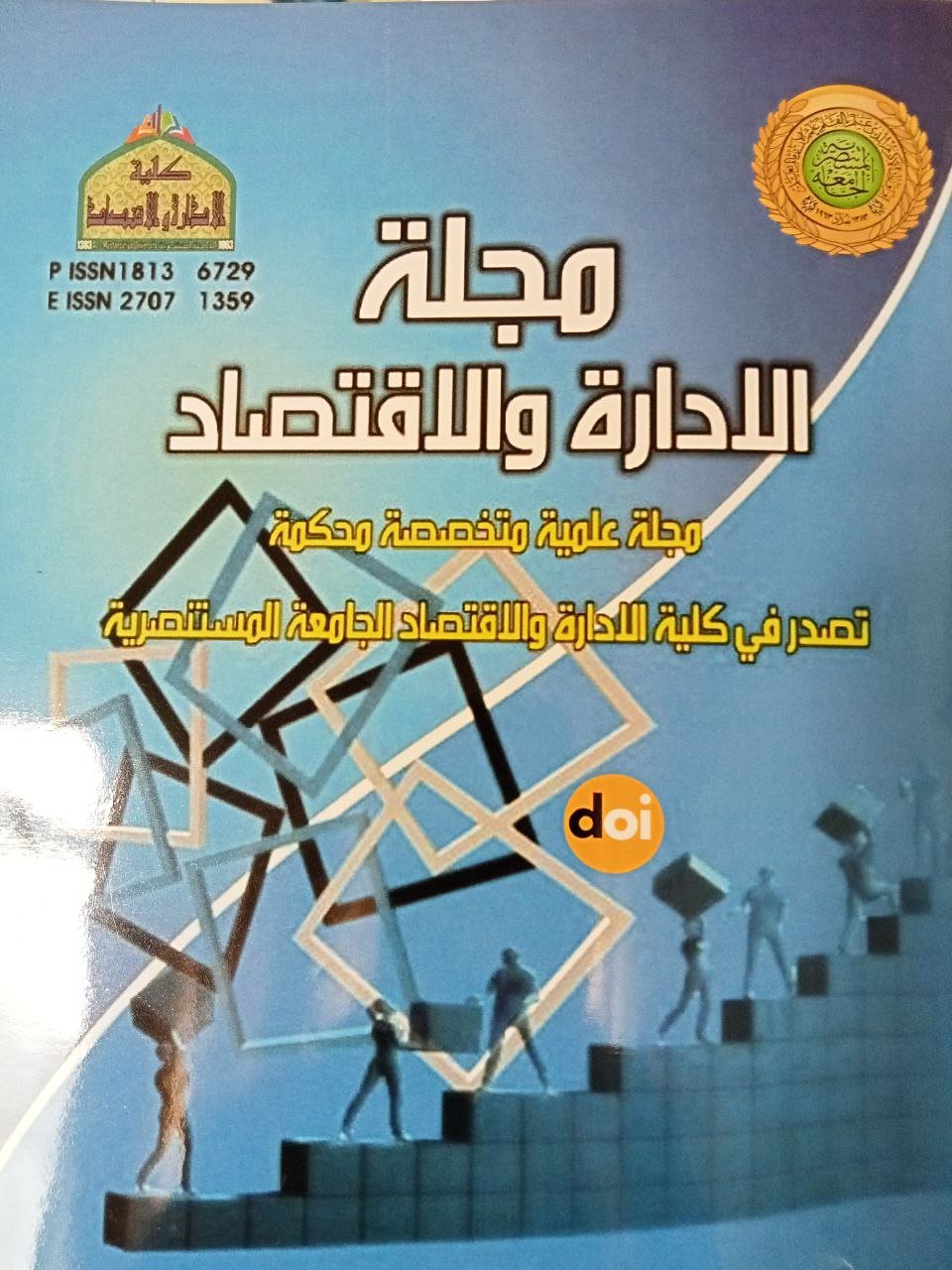