Using kernel regression to estimate the coefficients of the regression model with random error limits that are self-correlated with practical application
DOI:
https://doi.org/10.31272/jae.i132.673Keywords:
autocorrelation, kernel regression, local linear regression, Modified Mallo's criterionAbstract
The regression model is one of the models used for the purposes of interpreting the effect of a phenomenon or several phenomena on a particular phenomenon, by estimating the coefficients of the model, in addition to adopting the estimated model in making future predictions for the effect or effects of the interpreted phenomena on the response phenomenon. The regression model is built on several assumptions. If they are achieved, we can get estimates that have the desired characteristics. One of these assumptions is related to the limits of random error, as it is assumed that these limits are independent among them. Limits of random error and this problem has an impact on the least-squares estimates of the model coefficients, which leads to misleading results about the effect of the explanatory phenomena as well as for future predictions. There are several methods for estimating the coefficients of the regression model in light of this problem, including its parameters and non-parametric ones. One of the non-parametric methods is the kernel regression method, as the research aims to use this method to estimate the positional linear regression in estimating the regression model with self-correlated random error limits and depending on the modified Mallo criterion. The appropriate bandwidth parameter has been selected so that the bandwidth parameter has a clear influence on the estimation process and works to approximate and smooth the estimated curve from the real curve. A practical application was made on real data represented by the money supply and some factors affecting it, and through the use of the (Gaussian) and (Epanechnikv) kernel functions, the regression curve that represents the expected value of the money supply was estimated. The results of the estimation revealed that the kernel function (Gaussian) is the best in smoothing the regression function based on the comparison criteria MAE, RMSE, and MAPE, despite the convergence of the estimation results for the two kernel functions.
References
1- البنك المركزي العراقي ، المديرية العامة للإحصاء والابحاث ، مجموعات ، ونشرات احصائية سنوية للأعوام (2008- 2019) .
2- التميمي , زهرة حسن عباس و السعدون , فوزية غالب عمر و الثعلبي , ساهرة حسين زين (2014) " تحليل الانحدار " طبع في مديرية دار الكتب للطباعة و النشر جامعة البصرة .
3- جياس ، محمد عبد الواحد و محمد ، سحر قاسم (2016) "عرض النقد والعوامل المؤثرة فيه ودور البنك المركزي العراقي في السيطرة عليه بحث تطبيقي في البنك المركزي العراقي للمدة (2003-2014)" مجلة العلوم الاقتصادية والادارية العدد 93 ، المجلد 22 ، ص 380- 400 .
4- حمود , مناف يوسف . (2000) , " مقارنة مقدرات kernel اللامعلمية لتقدير دوال الانحدار " , رسالة ماجستير في الاحصاء , كلية الادارة والاقتصاد , جامعة بغداد .
5- الصفاوي، صفاء يونس ومتي ،نور صباح " (2011) تقدير دوال الانحدار اللامعلمي باستخدام بعض أساليب التمهيد". المجلة العراقية للعلوم الإحصائية )عدد خاص بوقائع المؤتمر العلمي الرابع لكلية علوم الحاسوب والرياضيات). مجلد 20, ص 371-390
6- عطیة ، عبد القادر محمد عبد القادر ،(2004)" الحدیث في الاقتصاد القیاسي بین النظریة والتطبیق، الدار الجامعیة، الاسكندریة.
7- كاظم، اموري هادي و مسلم ، باسم شليبه (2002)" القياس الاقتصادي المتقدم النظرية والتطبيق"، مطبعة دينا الامل، بغداد ، العراق .
8- متراس , بان احمد و محمد , الاء محمود (2013) " استخدام المقدر اللبي و أسلوب العنقدة بمعدل – k للتعرف على إيماءة اليد" مجلة الرافدين لعلوم الحاسوب و الرياضيات المجلد (10) العدد (1)
9- محمد ، شيخي (2011) "طرق الاقتصاد القياسي محاضرات وتطبيقات" دار الحامد، الطبعة الاولى، الجزائر.
10- Altman N., (1990), Kernel smoothing of data with correlated errors, J. Amer. Statist. Assoc. 85, pp. 749–759.
11- Aydin D. ,(2007) , A Comparison of the Nonparametric Regression Models using Smoothing Spline and Kernel Regression, World Academy of Science, Engineering and Technology 36, 253-257.
12- Chiu, S.T. ,(1989) , Bandwidth selection for kernel estimate with correlated noise, Statist. Probab. Lett. 8, pp. 347–354.
13- Fan. J. and Gijbels I. (1992) ,Variable Bandwidth and Local Linear Regression Smoothers, Ann. Stat. No-4, PP. 2002-2036.
14- Hart, J. and Wehrly T.E. , (1986), Kernel Regression Estimation Using Repeated Measurements Data, J. Amer. Statist. Assoc. 81, 1080-1088.
15- Lee, Young K and Mammen, Enno and Park , B U. ,(2010), Bandwidth selection for kernel regression with correlated errors, Statistics, 44:4, 327-340, DOI:10.1080/02331880903138452.
16- Loader , Clive R., (1999) , Bandwidth Selection: Classical or Plug-in , The Annals of Statistics , Vol. 27, No. 2, 415–438
17- Mallows, C. , (1973) , Some comments on Cp.Technometrics 15, 661-675.
18- Parzen E. ,)1962(, On estimation of a probability density function and mode , Ann. Stat. Vol.33, PP 1065-1076.
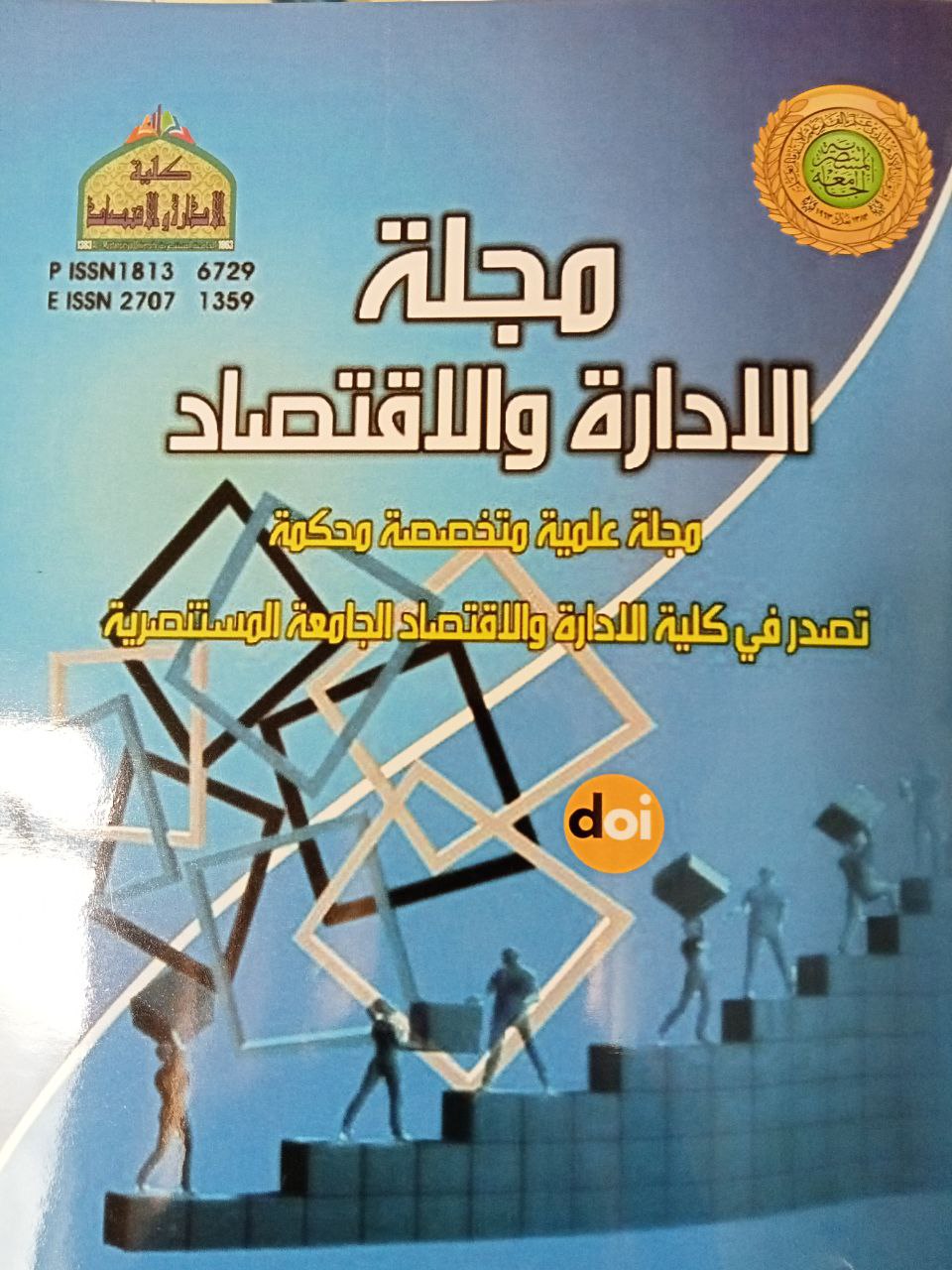
Downloads
Published
Issue
Section
License
The journal of Administration & Economics is an open- access journal that all contents are free of charge. Articles of this journal are licensed under the terms of the Creative Commons Attribution International Public License CC-BY 4.0 (https://creativecommons.org/licenses/by/4.0/legalcode) that licensees are unrestrictly allowedto search, download, share, distribute, print, or link to the full text of the articles, crawl them for indexing and reproduce any medium of the articles provided that they give the author(s) proper credits (citation). The journal allows the author(s) to retain the copyright of their published article.
Creative Commons-Attribution (BY)